How AI and Machine Learning Will Influence the eCommerce Industry
The growth of global eCommerce is mind-boggling. In 2017, retail eCommerce sales worldwide amounted to 2.3 trillion US dollars and eRetail revenues are projected to grow to 4.5 trillion US dollars in 2021 (according to a Statista report).
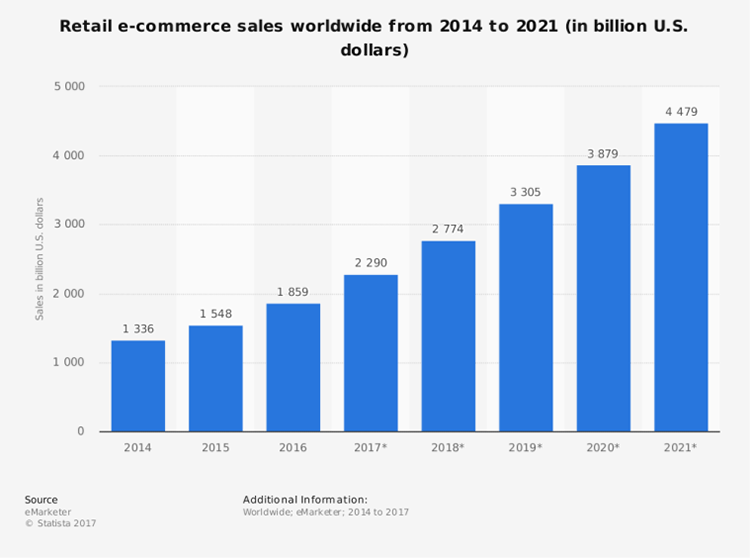
As the market has become more saturated and competitive, eCommerce businesses need to keep up with the latest technologies in order to succeed. By 2020, it will all boil down to machines being able to tackle much more efficient tasks that would normally require human input. Let’s take a closer look at what eCommerce businesses are doing at the moment.
The 3 marketing processes eCommerce businesses are now using
The pace of progress is ramping up so quickly in the eCommerce industry that businesses must adapt to consumer expectations as they are growing higher than ever. To create a personalised and holistic experience for their customers, there are 3 abilities eCommerce websites are using at the moment:
- They track (users, products, results and feedback)
- They understand (behavior, metrics, emotions, barriers)
- They adapt (offers, ads, website, prices)
With access to real-time information, analytics tools or interactive dashboards, retailers are gathering huge amounts of data getting closer to a point where campaigns and customer interactions can be made more relevant end-to-end. But how do they use all this data?
The 7 levels of using customers’ behavior
No data, no understanding, no personalisation - 10% of the current eCommerce startups
Big data, no understanding, no personalisation - Brick-and-mortar that just landed online and Mid-level eCommerce websites with no skilled data scientists
Big Data, manually understanding data, manually personalisation - eCommerce websites with a growth-oriented marketer that understands data through quantitative and qualitative analyses and creates rules to personalise and customise the customer experience
Big Data, automatically recognizing patterns & creating clusters, rule-based personalisation - mid-level eCommerce players with a data-driven approach and an optimisation team that identifies patterns and creates clusters from 1st party data, which are then manually personalised through rules
Big data, automatically recognizing patterns & creating clusters, manual personalisation - eCommerce websites with skilled data scientists and growth teams (ASOS, Booking.com) that gather big data, automatically recognise patterns and creates clusters from 1st, 2nd & 3rd party data.
Smart Big Data, automatically track & manually understand the unified customer profile - top 1% eCommerce players - Amazon
Automatically track behavior, automatically understand, automatically personalize the offer - Businesses that automatically track behavior, understand and unify the customer profiles and personalise to each profile on all the channels with no human intervention.
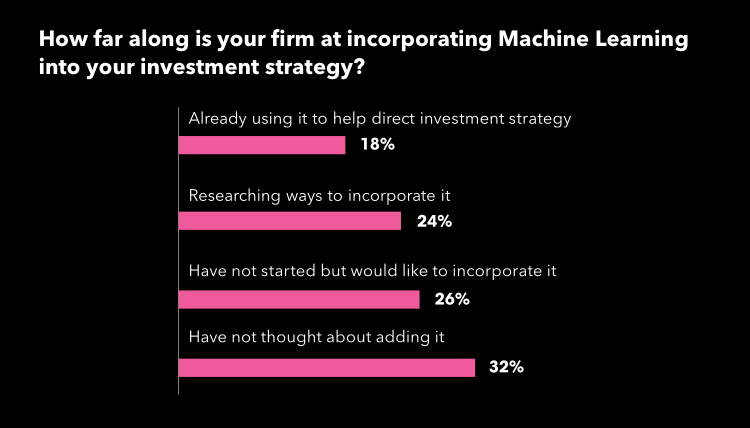
How machine learning will get eCommerce businesses one step closer to relevance at scale
To parse huge amounts of data and draw relevant conclusions, businesses are increasingly using artificial intelligence to extract patterns, gain a greater understanding of what customers actually want, and build genuine connections with them. One of AI’s most impressive features, machine learning and its predictive nature, is the process of predicting future behavior based on past experience.
Basically, computers and their models learn from data and adapt independently as more data is fed to them. But how can those predictions help increase conversion rates, lead to more conclusive testing and generate machine-powered analytics?
More targeted advertising
Machine learning technology will be critical to advertising. It can help marketers analyse countless signals in real time to anticipate consumer needs and reach them with more tailored ads. Predictive analytics uses machine learning to predict future outcomes based on behavioural patterns seen in historical data. Campaigns can target audience segments based on a huge number of behavioural signals, ads can be personalised to be more relevant in the context of the user, and bids can be optimised based on user data — all faster and with higher success rates than humans can manually accomplish.
Predictive advertising is changing the landscape from prospecting or pretargeting, to retargeting, ad mediation and campaign optimization. Here’s how predictive advertising looks like:
Smarter Search Engines
This is probably one of the areas with the highest impact from machine learning algorithms. The semantic search engines are using both Natural Language Processing and Artificial Intelligence to understand long search terms (like women’s blue jacket under $100). Machine Learning techniques boost the ability of internal website searches to understand the customer's intent (i.e. identify the typos, recognise synonyms, show related relevant products) as opposed to focusing only on specific keywords.
The field of natural language processing is shifting from statistical methods to neural network methods. Deep learning methods are achieving state-of-the-art results on language modelling, speech recognition, caption generation, machine translation, document summarisation and question answering.
Definitely one of the most hyped technologies introduced in the last few years, Voice Search is the fastest growing search type worldwide. To showcase this growth bare in mind that, last year, 20% of all mobile queries were voice searches. 50% of all searches will be voice searches by 2020 according to comscore and about 30% of searches will be done without a screen by 2020.
AI and machine learning are all about image search and voice search. Those capabilities and technologies are going to be core to search and that is where search and consumer engagement is headed.
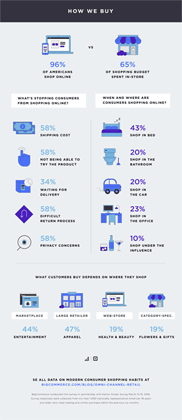
More relevant customer behaviour predictions
So much of the potential for using data generated by a website is left unexplored. Detailed behavior data insights are becoming accessed by more and more businesses, but knowing how to use those insights and how to automatise the process is key.
Through machine learning techniques, businesses will be able to create a model, use data collected about customer behavior to date, and try to predict the likelihood of an event occurring. This will translate into: intuitive search and display, smart recommendations engines, processing customer service inquiries, and more accurate Content Based Recommendation (by matching user’s interests with description and attributes of items). The better the predictions, the better the user experiences on eCommerce websites, so the better the conversion rates.
Predictive analytics
A lot of marketing campaigns have operated on assumptions, but we can now apply this principle with greater accuracy and accountability. Where AI comes into its own in this field is in its ability to identify broader patterns that humans simply would not see.
We select areas for investigation based on what we believe to be safe assumptions, but AI can identify the impact of obscure patterns that humans would not. This helps businesses improve their conversion rate, but the implications are much wider than that. Predictive analytics allows companies to set pricing strategies based on consumer expectations and competitor benchmarks. It allows retailers to anticipate demand, and therefore ensure they have the right level of stock for each product.
The evidence of this revolution is already all around us. Google has been using this technology for some time already, through its Smart Goals product in Analytics. This is only the beginning, however. Google’s DeepMind team has just created an AI that is capable of planning for the future and considering different outcomes before acting. More than that (and this is not recent news, but it’s still mind-boggling) Amazon has filed a patent for a shipping system designed to cut delivery times by predicting what buyers are going to buy before they buy it — and shipping products in their general direction, or even right to their door, before the sales click even (or ever) falls. Good news for any business that also sells via Amazon.
How can businesses integrate machine learning into their strategy?
- The right questions - The best projects begin with a sound hypothesis to test.
- The right data - The next stage after defining the questions is figuring out what data is available to you and whether it will be sufficient to answer your questions.
- The right technology - Data is created at such a rate that we require ever-improving technological capabilities just to capture, store, and make sense of it.
- The right people - Without the right people, it’s very difficult to pose the right questions. It is also difficult to know what technology to use and which is the most suitable option for you.
For all of the talk of AI replacing people, it really just revealed the importance of finding the right people to make the most of the new opportunities it creates.